It's 10PM. Do You Know Where Your Training Data Is?
With the whipsaw rate of the evolving landscape of generative artificial intelligence (GenAI), the age-old adage "garbage in, garbage out" takes on a whole new level of significance.
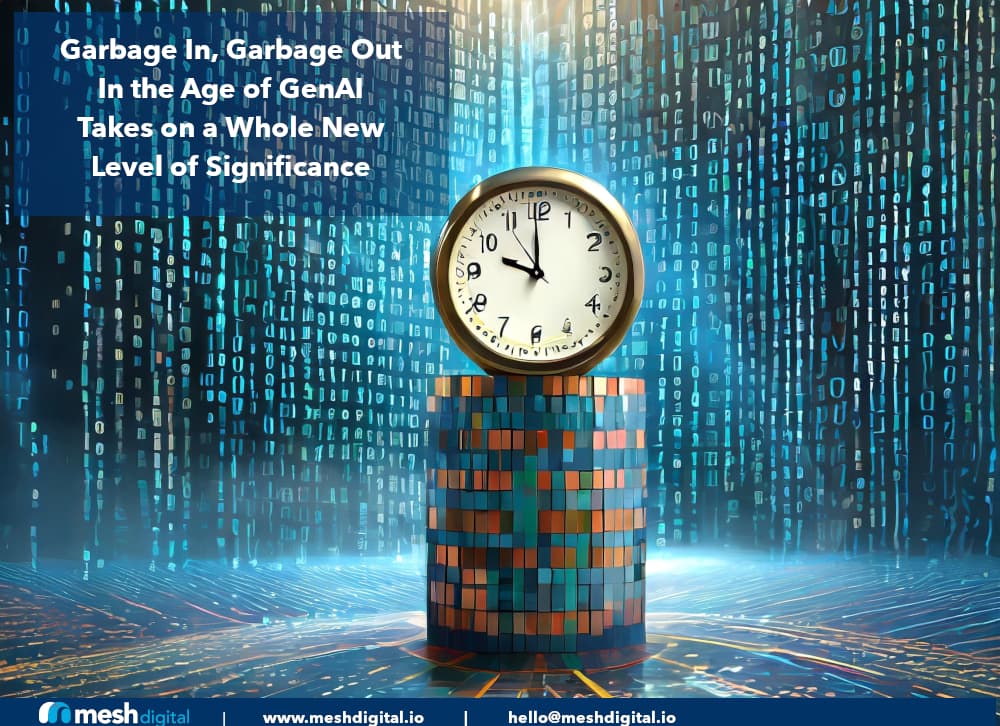
With the whipsaw rate of the evolving landscape of generative artificial intelligence (GenAI), the age-old adage "garbage in, garbage out" takes on a whole new level of significance. As organizations race to harness the power of GenAI platforms, the quality and integrity of their training data have become paramount concerns. Just as a master chef's culinary creations are only as good as their ingredients, the performance and trustworthiness of GenAI systems hinge on the data they are fed.
This article delves into the often overlooked, yet crucial, aspect of Training Data Management for GenAI platforms. We'll briefly explore the potential pitfalls of haphazard data handling, the ethical and regulatory implications, and the strategic considerations organizations must embrace to ensure their GenAI initiatives are built on a solid foundation of high-quality, responsible, and trustworthy data whose data lineage can be traced.
The Hidden Risks of Poorly Managed Training Data
The allure of GenAI's potential is undeniable – from automating repetitive tasks and enhancing customer experiences to uncovering groundbreaking insights and driving innovation. However, this transformative power comes with a caveat: the quality of the output is inherently tied to the quality of the input data.
Poorly curated, biased, or incomplete training data can lead to a myriad of issues, ranging from inaccurate predictions and flawed decision-making to perpetuating harmful biases and compromising user trust. In the worst-case scenarios, such data mismanagement can result in legal and reputational consequences for organizations, as well as societal harm.
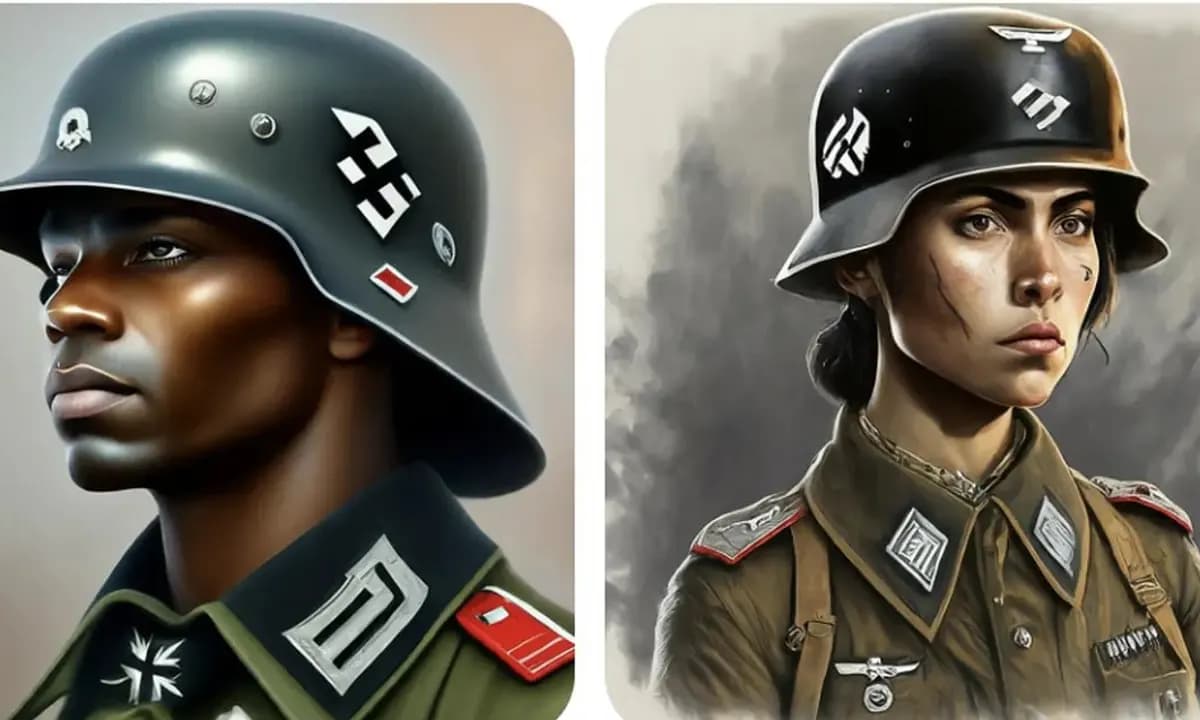
For instance, imagine a GenAI system trained on a dataset skewed towards certain demographics or viewpoints. This shouldn’t be hard to believe, considering some well known GenAI gaffes have unearthed skewed algorithms. Generating unfortunate negative PR for firms like Google with their Gemini platform, which has found to generate factually inaccurate outputs, as seen in the above images. Such a system could inadvertently propagate biases, leading to unfair treatment of underrepresented groups, or creating factually incorrect information, or the amplification of harmful stereotypes. Similarly, a GenAI platform trained on outdated or incomplete data could produce inaccurate outputs, jeopardizing critical business decisions or undermining customer experiences.
These risks underscore the need for a robust, proactive, and holistic approach to training data management, one that prioritizes data quality, ethics, and responsible AI practices from the outset.
The Five Pillars of Responsible Training Data Management
To mitigate the risks associated with poor training data and unlock the full potential of GenAI, organizations must adopt a comprehensive framework that addresses at a minimum the following five pillars:
- Data Quality & Curation: Establishing rigorous processes for data collection, cleansing, and validation is paramount. This involves implementing quality checks, addressing data gaps or inconsistencies, and ensuring that the training data accurately represents the real-world scenarios in which the GenAI platform will operate.
- Bias Mitigation: Identifying and mitigating biases within training data is crucial to prevent the perpetuation of harmful stereotypes or discrimination. Organizations should leverage techniques such as bias audits, data augmentation, and adversarial training to address potential biases proactively.
- Data Privacy & Security: With the increasing prevalence of personal and sensitive data in training datasets, robust data privacy and security measures are essential. This includes adhering to applicable data protection regulations, implementing strict access controls, and ensuring the secure storage and handling of training data throughout its lifecycle (part of a data lineage practice).
- Ethical & Regulatory Compliance: Beyond legal obligations, organizations must embrace ethical principles and guidelines for responsible AI development and deployment. This involves establishing governance frameworks, conducting ethical risk assessments, and ensuring transparency and accountability in the use of training data.
- Continuous Monitoring & Improvement: As GenAI systems are deployed and interact with the real world, continuous monitoring and improvement of training data is crucial. Organizations should establish feedback loops to identify and address data drift, incorporate new data sources, and refine their models to maintain high levels of performance and trustworthiness over time.
Key Considerations for Responsible GenAI Development
In addition to the five pillars, organizations must also navigate a range of critical considerations to ensure the responsible and sustainable development of GenAI systems including, but not limited to:
- Interpretability & Explainability: GenAI models, particularly those based on deep learning architectures, can often be opaque and difficult to interpret. Organizations must prioritize the development of interpretable and explainable models, ensuring transparency and accountability in decision-making processes.
- Robustness & Resilience: GenAI systems must be designed to be robust and resilient against adversarial attacks, data corruption, or unexpected edge cases. This involves implementing techniques such as adversarial training, data augmentation, and rigorous testing to improve model resilience.
- Environmental Sustainability: The training and deployment of large-scale GenAI models can have significant environmental impacts due to their computational and energy requirements. Organizations must prioritize energy-efficient computing, leverage renewable energy sources, and explore techniques for model compression and optimization to reduce their carbon footprints.
- Economic & Organizational Resilience: Adopting GenAI technologies can disrupt existing business models and organizational structures. Organizations must carefully assess the economic implications, cultivate a culture of agility and adaptability, and ensure the long-term viability of their operations in the face of technological disruption.
- Cultural Diversity & Inclusivity: To harness the full potential of GenAI and mitigate biases, organizations must embrace cultural diversity and inclusivity, while keeping a pragmatic lens. This involves incorporating diverse perspectives, experiences, and backgrounds into training data curation processes, model development teams, and decision-making frameworks.
- Stakeholder Engagement & Trust-Building: Fostering trust and acceptance among stakeholders, including customers, employees, and the broader public, is crucial for the successful adoption of GenAI technologies. Organizations should prioritize transparency, education, and open communication to address concerns and build trust in their GenAI initiatives.
By addressing these considerations, organizations can navigate the complexities of responsible GenAI development, mitigating risks, and unlocking the full potential of these transformative technologies while upholding ethical principles and societal well-being.
Tuning GenAI Models
Warranting a deeper dive article in and of itself (🤔), there are several evolving techniques for fine-tuning, or interrogating, or even "conversing" with GenAI models to steer them in the right direction. These tuning opportunities are within the span of control for most enterprises, with some prominent current techniques detailed here:
- Prompt Engineering: LangChain is one of the APIs that some teams are using to semi-automate this process, while providing additional transparency, metrics, and the ability to manage the GenAI models.
- Retrieval Augmented Generation (RAG): involves reaching into structured data, based on its inputs or outputs from LLMs with the same intent - to provide curated / trusted information back to the LLM in the form of prompts or additional embeddings (the representation of text in vector space on which the LLMs do their math). This is currently done with relational databases and knowledge graphs.
- R&D & Emerging Rails Techniques: There are active works in progress to improve the training of LLMs as well as improvements to the self-checking mechanisms on the back end to help keep the models "on the rails," providing a set of guardrails for the LLM using combinations of structured and unstructured data.
- Unifying LLMs & Knowledge Graphs: Efforts are underway to integrate capabilities of LLMs and Knowledge Graphs to achieve synergies between bottom-up learning and top-down knowledge-base reinforcement.
- LLM-Powered Unstructured Analytics: Arguments that current RAG approaches are insufficient and brittle, and that a more flexible approach inspired by the tenets that made relational databases successful can aid in turning GenAI models.
- Bias Effects And Notable Generative AI Limitations (BENGAL): Efforts sponsored by the U.S. Office of the Director of national Intelligence, Intelligence Advanced Research Projects Activity (IARPA) with the goal to understand large language model (LLM) threat modes, quantify them and to find novel methods to address threats and vulnerabilities or to work resiliently with imperfect models. IARPA seeks to develop and incorporate novel technologies to efficiently probe LLMs to detect and characterize LLM threat modes and vulnerabilities, including of course biases.
- Red Teaming: Using a manual, human-driven verification, and assurance capabilities. With this route, the model is verified and checked for possible vulnerabilities and risks.
- Agent Based Models: Human agents, acting to verify and govern the LLM model interactions, while ensuring not just safety, but that preferred outcomes are achieved.
Conclusion: Redefining the Future of GenAI
As the GenAI revolution continues to reshape industries and redefine the boundaries of what's possible, the quality and integrity of training data have emerged as critical success factors. By adopting a proactive and holistic approach to training data management, organizations can unlock the full potential of GenAI while mitigating risks and fostering trust among stakeholders.
Embracing the five pillars of responsible training data management – data quality and curation, bias mitigation, data privacy and security, ethical and regulatory compliance, and continuous monitoring and improvement – is essential for building robust, trustworthy, and impactful GenAI systems.
Moreover, organizations must navigate a range of considerations, including interpretability and explainability, robustness and resilience, environmental sustainability, economic and organizational resilience, cultural diversity and inclusivity, and stakeholder engagement and trust-building.
By addressing these challenges head-on, organizations can position themselves at the forefront of the GenAI revolution, redefining the future of innovation, decision-making, and value creation while upholding the highest standards of responsibility and ethics.
In the end, the success of GenAI initiatives hinges not only on cutting-edge algorithms and computational power but also on the often-overlooked foundation of high-quality, responsible, and trustworthy training data. So, at 10PM or any other time, organizations must always know where their training data is and ensure it meets the highest standards of integrity, ethics, and accountability.
References:
- Barocas, S., Hardt, M., & Narayanan, A. (2019). Fairness and machine learning. fairmlbook.org.
- Brundage, M., Krueger, G., Haselager, W. F., & van de Poel, I. (2022). AI ethics: A grand challenge for ethics and society. AI and Ethics, 123. https://doi.org/10.1007/s43681022001653
- Crawford, K., & Calo, R. (2016). There is a blind spot in AI research. Nature, 538(7625), 311313. https://doi.org/10.1038/538311a
- Floridi, L., & Cowls, J. (2019). A unified framework of five principles for AI in society. Harvard Data Science Review, 1(1). https://doi.org/10.1162/99608f92.8cd550d1
- Gebru, T., Morgenstern, J., Vecchione, B., Vaughan, J. W., Wallach, H., Daumeé III, H., & Crawford, K. (2021). Datasheets for datasets. Communications of the ACM, 64(12), 8692. https://doi.org/10.1145/3458723
- Mitchell, M., Wu, S., Zaldivar, A., Barnes, P., Vasserman, L., Hutchinson, B., ... & Gebru, T. (2019). Model cards for model reporting. In Proceedings of the conference on fairness, accountability, and transparency (pp. 220229).
- Raji, I. D., Smart, A., White, R. N., Mitchell, M., Gebru, T., Hutchinson, B., ... & Barnes, P. (2020). Closing the AI accountability gap: Defining an endtoend framework for internal algorithmic auditing. In Proceedings of the 2020 Conference on Fairness, Accountability, and Transparency (pp. 3344).
- Zook, M., Barocas, S., Boyd, D., Crawford, K., Keller, E., Gangadharan, S. P., ... & Narayan, A. (2017). Ten simple rules for responsible big data research. Data & Society, 30, 130.