The Power of Going Small: How Small Language Models are Driving Competitive Advantage in AI
In the race for AI supremacy, it’s easy to assume that bigger is always better. With large language models (LLMs) like GPT-4o and others dominating headlines, many CDOs are left wondering: Is investing in these behemoths the only path to success? Enter small language models (SLMs).
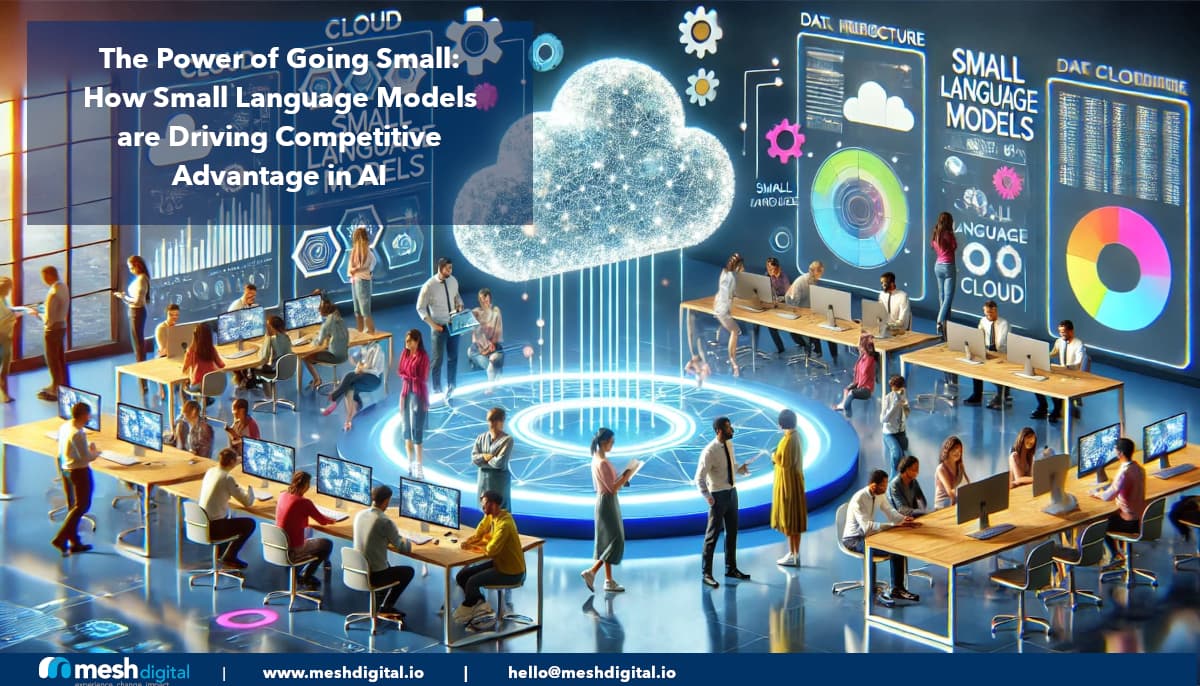
In the race for AI supremacy, it’s easy to assume that bigger is always better. With large language models (LLMs) like GPT-4o, Google's Gemini, and Anthropic's Claude (amongst many others) and others dominating headlines, many Chief Data Officers (CDOs) are left wondering: Is investing in these behemoths the only path to success? Or, is there a smarter, more strategic way to approach AI?
Enter small language models (SLMs). While LLMs are rapidly becoming commoditized and accessible to the masses. SLMs are quietly proving that "going small" offers distinct advantages. Here’s why CDOs should be thinking twice before betting their AI strategy solely on the largest models available.
Efficiency Over Extravagance
LLMs are computational juggernauts, requiring massive hardware, energy, and time to train. For many organizations, this leads to bloated infrastructure costs and inefficiencies (or big service provider bills). A study by Patterson et al. (2021) highlights that training large AI models is significantly more energy-intensive than smaller alternatives. But what happens when the performance gains of these models hit diminishing returns? This is where SLMs shine.
SLMs offer a much more efficient use of resources. They require less data, fewer parameters, and are computationally cheaper to train and deploy. While LLMs may have their place in more generalized tasks, SLMs are often better suited to solving domain-specific problems with greater precision. As Andrew Ng aptly points out, "It’s not about the size of the model; it’s about the size of the data" (Ng, 2020). In specialized applications, SLMs can outperform their larger cousins without the resource drain nor massive expenditures.
Faster Time to Market: Flexibility and Adaptation
In a world where speed is critical, LLMs especially pre-trained ones can be slow to deploy. Their vast size means they require significant tuning, retraining, and fine-tuning to be effective in real-world applications. This translates into longer time-to-market, something that can be a dealbreaker in industries where first or fast-mover advantage is key.
SLMs, by contrast, are nimble. They can be quickly retrained on smaller datasets, fine-tuned for specific tasks, and adapted to new situations with minimal overhead. This ability to iterate and deploy rapidly gives organizations a crucial edge, especially when dealing with evolving customer demands or fast-moving market conditions (Brown et al., 2021). Competitive advantage often lies in agility, and SLMs provide exactly that.
Customization Without the Compromise
LLMs are impressive generalists. They can write poetry, code websites, and answer trivia, all in one breath. But this flexibility can also be their Achilles' heel. By trying to do everything, LLMs often lack the deep specialization required for niche tasks. Many CDOs are starting to realize that domain-specific tasks don’t require a jack-of-all-trades approach, but a tool that’s laser-focused.
SLMs, on the other hand, are perfect for hyper-focused customization. By training smaller models on targeted datasets, organizations can achieve greater accuracy in specific applications, be it legal document analysis, healthcare diagnostics, or customer sentiment analysis. As emphasized by Bommasani et al. (2021), smaller models allow for a deeper alignment with business-specific needs, making them a valuable asset for organizations that want to fine-tune AI to their exact specifications.
Data Privacy and Security: The CDO’s Dilemma
With data privacy regulations tightening worldwide, CDOs (and CISOs) are increasingly aware of the challenges associated with using LLMs. LLMs often require vast amounts of public data for training, but they can also be vulnerable to inadvertent leakage of sensitive information, especially in industries like finance and healthcare (Bommasani et al., 2021). The risk of exposing proprietary or confidential data through massive-scale models is a serious concern.
SLMs, by contrast, allow for tighter control over data privacy (making CISOs happier). Since they can be trained on smaller, more curated datasets, organizations can ensure that sensitive information is handled with greater care. Moreover, SLMs are often deployed in more controlled environments, reducing the risk of unintended data exposure. For CDOs focused on compliance and data governance, SLMs offer a pathway to AI-driven innovation that doesn’t compromise security.
Cost Efficiency: Small Models, Big Savings
Let’s talk dollars and cents (or pounds and pence across the pond). LLMs are expensive—not just to train, but to maintain. The hardware requirements alone can be prohibitive for smaller enterprises. But even for large organizations, the cost-benefit analysis is becoming increasingly clear: Why sink millions into a massive model when a smaller one (or several, specialized smaller ones) can deliver similar, if not better, results for certain tasks?
SLMs not only save on initial training costs but also reduce the need for expensive cloud or on-premise infrastructure and their ongoing maintenance. According to research by Strubell et al. (2019), using smaller models can cut down on both financial and environmental costs by an order of magnitude, making AI more sustainable and accessible to businesses that don’t have bottomless pockets.
LLMs Are Becoming Commoditized
As more players enter the AI space, LLMs are quickly losing their “special sauce.” OpenAI, Google, Microsoft, and others are offering pre-trained LLMs through APIs and platforms, making them widely available to anyone willing to pay for access. In essence, LLMs are becoming commoditized. The same model that powers one company’s chatbot could be powering their competitor’s virtual assistant.
SLMs, on the other hand, provide a unique competitive advantage. By training smaller, proprietary models that are tailored to specific business needs, organizations can create AI solutions that are difficult for competitors to replicate. This level of customization allows businesses to stand out in an increasingly crowded AI landscape, offering differentiated products and services.
The Future of AI is Hybrid
While SLMs are gaining ground, it’s important to acknowledge that there isn’t a one-size-fits-all solution in AI. The future lies in hybrid approaches, where CDOs can leverage the strengths of both large and small models, depending on the task at hand.
For tasks that require broad generalization and knowledge, LLMs will still play a role. But for tasks that demand precision, efficiency, and cost-effectiveness, SLMs are becoming the go-to solution. By strategically using the right tool for the job, organizations can maximize their AI investments and drive sustainable competitive advantage.
Conclusion: Why Small is the New Big in AI
In the ever-evolving AI landscape, bigger doesn’t always mean better. As LLMs become commoditized and accessible to the masses, the real opportunity for competitive differentiation lies in the power of going small. SLMs offer organizations the ability to be more efficient, agile, and focused, all while maintaining control over data privacy and cost.
For CDOs looking to navigate this AI revolution, the key takeaway is simple: Don’t get caught up in the size of the model. Focus on the strategy behind it. Because, in the world of AI, it’s the small, smart decisions that drive the biggest impact.
Lean More About SLMs
Check out our next article in this series called "Small Language Models (SLMs), Big Impact: Navigating the Best AI Solutions for Your Business in 2024."
References
Bommasani, R., Alecta, D., Ling, J., et al. (2021). On the opportunities and risks of foundation models. arXiv preprint arXiv:2108.07258.
Brown, T. B., Mann, B., Ryder, N., et al. (2021). Language models are few-shot learners. Advances in Neural Information Processing Systems, 33, 1877–1901.
Ng, A. (2020). The batch: Data-centric AI development. Retrieved from https://www.andrewng.org/
Patterson, D., Gonzalez, J., Le, Q. V., Liang, C., Munguia, L., Rothchild, D., & Dean, J. (2021). Carbon emissions and large neural network training. arXiv preprint arXiv:2104.10350.
Strubell, E., Ganesh, A., & McCallum, A. (2019). Energy and policy considerations for deep learning in NLP. arXiv preprint arXiv:1906.02243.