Structuring a Framework for Small Language Model (SLM) Adoption in Highly Regulated Markets: A Pragmatic Approach
Adopting AI in regulated sectors like finance, healthcare, and government is a delicate balance. Compliance, data security, and transparency are non-negotiable, making innovation feel more like walking a tightrope than sprinting forward—especially when AI plays a central role.
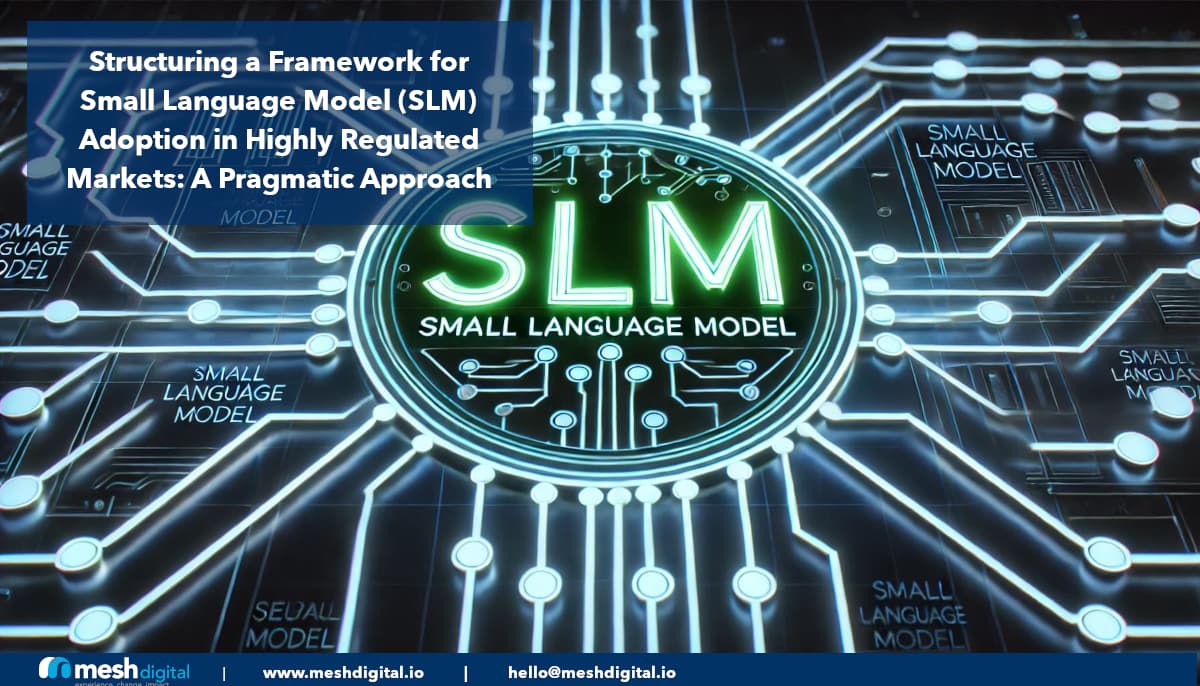
When navigating AI adoption in highly regulated markets like Finance, Healthcare, and the Insurance sectors, "Innovation" can feel more like a tightrope walk than an open sprint. Regulatory frameworks demand compliance, data security, and transparency—none of which can be compromised, especially when AI is involved. Yet, despite these constraints, adopting small language models (SLMs) can provide organizations with the agility and precision they need while ensuring they remain within the bounds of compliance.
At Mesh Digital LLC, we recognize that this isn’t just about deploying AI for AI’s sake; it’s about structuring an adoption framework that balances innovation with regulation. Building on our prior post on the topic of "The Power of Going Small: How Small Language Models are Driving Competitive Advantage in AI." As well as, steered by our "Mesh Digital’s Adaptive AI Framework: A Best Practice Approach to Agile AI Deployment." Here's how we help our clients stay ahead in the AI game without losing their footing.
Understanding Regulatory Compliance: Start with Data Governance
The first pillar of any AI strategy in a regulated market is robust Data Governance. For SLMs (and LLMs) to be effective, CDOs and IT leaders must ensure that their data practices align with internal governance and risk policies, as well as external regulations like GDPR, HIPAA, HITECH or the alphabet soup of other regulations.
One key advantage of SLMs is that they can be trained on smaller, more controlled datasets. This is a significant differentiator from larger models, which often require vast amounts of data that could inadvertently include sensitive or non-compliant information. By working with SLMs, organizations can tighten the scope of their data training pipelines, mitigating the risks associated with data breaches or violations.
Mesh Digital's Differentiation:
At Mesh Digital LLC, we guide our clients to build a Data Trust Framework that emphasizes granular control, traceability, and governance of datasets from day one. This ensures that the datasets used for model training are not only compliant but also specifically curated to meet regulatory demands without sacrificing accuracy or performance.
Framework for Risk Management: Explainability and Accountability
AI models, especially in regulated sectors, need to be explainable. With large language models, the “black box” problem is a common concern—how do we understand or explain the decisions made by these massive systems? SLMs offer a solution here. Their smaller, more specialized nature makes it easier to interpret their outputs, which is vital for accountability, especially when regulatory scrutiny is high.
SLMs allow for simpler auditing, and their outputs can be aligned with compliance reports, making it easier to track decisions and ensure regulatory standards are met. Organizations need to adopt a risk management framework that includes regular AI audits, transparency in model decision-making, and clear documentation of how decisions are derived.
Mesh Digital's Differentiation:
Our Compliance-First AI Strategy integrates built-in checkpoints for explainability, offering transparent decision trees for how AI conclusions are drawn. This feature is especially valuable for clients in Finance and Healthcare, where both regulatory bodies and stakeholders require clear documentation of every AI-driven decision.
Operationalizing AI in Regulated Markets: Control and Scalability
Deploying SLMs in production environments within regulated industries requires a careful balance between control and scalability. Since SLMs are less resource-intensive, they can be operationalized faster and with fewer infrastructure demands, allowing organizations to quickly scale up AI operations in a controlled, compliant manner.
Organizations can build modular AI systems where specific SLMs are used for distinct tasks—each model can be deployed in controlled environments with its own set of regulations and checks. For example, a healthcare provider might use one SLM for patient data processing and another for medical diagnostics, ensuring that both models comply with industry-specific requirements.
Mesh Digital's Differentiation:
We work with clients to develop AI Deployment Pods—small, highly controlled environments where SLMs are deployed in sandboxed conditions before being integrated into broader operational systems. These pods allow for rigorous testing against regulatory standards while offering scalable deployment strategies.
Customization for Regulatory Edge: Domain-Specific Training
One of the strengths of SLMs lies in their ability to be highly customized for specific tasks, particularly in industries with complex regulatory requirements. By tailoring SLMs to industry-specific datasets, organizations can achieve better accuracy and compliance than they might with generalized models. The flexibility of SLMs makes it easier to control data inputs and outputs, ensuring that regulatory standards are embedded in every step of the AI process.
For example, in the Banking Sector, SLMs can be trained to detect regulatory anomalies in financial transactions, such as money laundering red flags or insider trading patterns. The ability to tailor a model to detect specific regulatory requirements gives organizations a clear advantage in compliance.
Mesh Digital's Differentiation:
At Mesh Digital we help clients by developing Regulatory-Focused AI Models that don't lose site that they need to meet and fulfill business needs and although the governance needs to be robust, it needs to as frictionless as possible (which isn't all that easy to be candid). These SLMs are trained with domain-specific regulatory datasets, ensuring the models are not only optimized for performance but also compliance. This tailored approach reduces the risk of non-compliance and allows our clients to remain agile in highly regulated markets.
Continuous Learning and Adaptation: Monitoring and Feedback Loops
Regulated markets are constantly evolving, with new laws, standards, and guidelines regularly introduced. AI models must keep pace with these changes, and that’s where continuous learning plays a vital role. Organizations must implement feedback loops that allow SLMs to adapt to regulatory changes over time.
SLMs, once again due to their smaller size, are more adaptable and can be re-trained more efficiently when new regulatory guidelines emerge. This reduces downtime and ensures that organizations can remain compliant without significant delays in AI model retraining.
Mesh Digital's Differentiation:
Our Adaptive AI Framework integrates continuous monitoring tools that assess the performance of SLMs in real time. This framework not only identifies potential compliance risks before they escalate but also ensures that models can be updated and retrained seamlessly as new regulations arise.
Conclusion: Structured Simplicity for Competitive Advantage
The adoption of SLMs in highly regulated markets requires a strategic, structured approach that balances innovation with compliance. By focusing on Data Governance, Risk Management, Operational Control, Customization, and Continuous Learning (both people and tech!), organizations can deploy AI solutions that not only meet regulatory requirements but also drive competitive advantage.
At Mesh Digital LLC, we believe in pragmatic, tailored strategies that enable our clients to innovate within the bounds of regulation, ensuring that the power of SLMs is fully harnessed while maintaining compliance every step of the way.
References
Bommasani, R., Alecta, D., Ling, J., et al. (2021). On the opportunities and risks of foundation models. arXiv preprint arXiv:2108.07258.
Strubell, E., Ganesh, A., & McCallum, A. (2019). Energy and policy considerations for deep learning in NLP. arXiv preprint arXiv:1906.02243.